Lemmy 0.19 updates
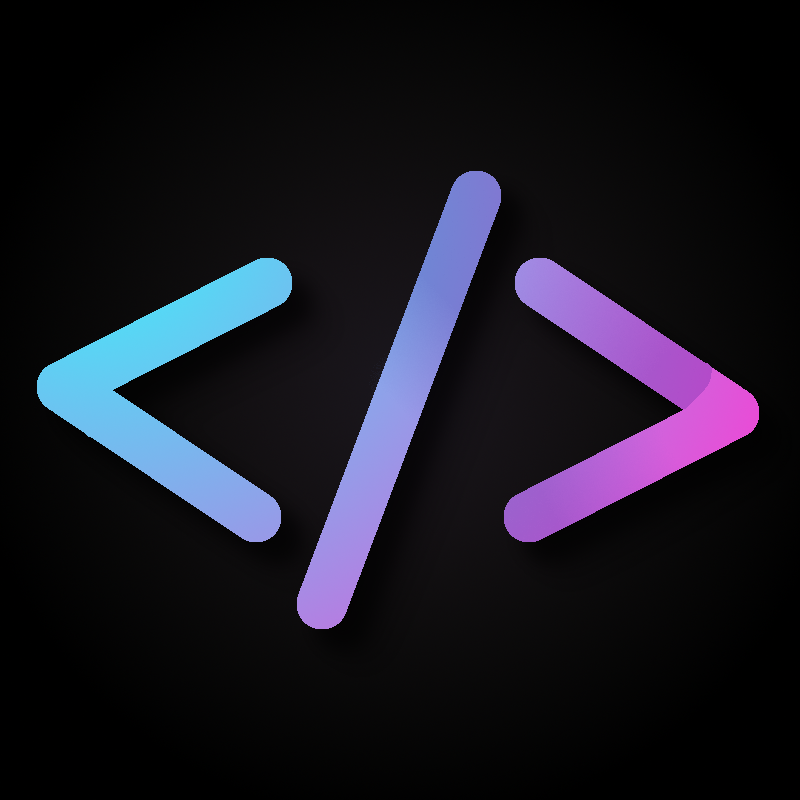
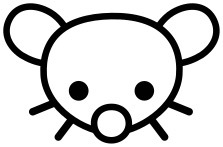
Although your current role wouldn’t seem very senior at a large organizational, “senior“ is a relative term and at this company it seems like you are the engineer with ownership responsibilities over the end-to-end software development of a production system. So it might still be reasonable to use a senior title if there are other benefits
It’s probably not going to work as a defense against training LLMs (unless everyone does it?) but it also doesn’t have to — it’s an interesting thought experiment which can aid in understanding of this technology from an outside perspective.
Agreed. My copy lost this documentation link in the original which gives more detail about the horizontal scaling: https://join-lemmy.org/docs/administration/horizontal_scaling.html.
It seems really straightforward (which is a good thing), each backend Lemmy_server handles incoming requests and also pulls from a shared queue of other federation work.
Collage sounds really interesting , will check it out. Another variation on bloom filter I recently learned about is count-min-sketch. It allows for storing/incrementing a count along with each key, and can answer “probably in set with count greater than _”, “definitely not in set”.
Thanks for adding more detail on the DB use-cases!
This data structure uses a 2-dimensional array to store data, documented in this scala implementation: https://github.com/twitter/algebird/blob/develop/algebird-core/src/main/scala/com/twitter/algebird/CountMinSketch.scala. I’m still trying to understand it as well.
Similar to your idea, I had thought that by using k bloom filters, each with their own hash function and bit array, one could store an approximate count up to k for each key, which also might be wasteful or a naïve solution.
PDF link: http://www.eecs.harvard.edu/~michaelm/CS222/countmin.pdf
I haven’t used them in Spark directly but here’s how they are used for computing sparse joins in a similar data processing framework:
Let’s say you want to join some data “tables” A and B. When B has many more unique keys than are present in A, computing “A inner join B” would require lots of shuffling if B, including those extra keys.
Knowing this, you can add a step before the join to compute a bloom filter of the keys in A, then apply the filter to B. Now the join from A to B-filtered only considers relevant keys from B, hopefully now with much less total computation than the original join.
I agree with how you characterized it and the term “ai engineer” didn’t resonate with me as defined by the author. If such an engineer doesn’t need to know about the data involved (“nor do they know the difference between a Data Lake or Data Warehouse”) then I don’t think they will be able to ship an AI/ML product based on data.
New titles can be helpful for sorting out different roles with some shared skillsets such as the distinction which emerged between Data Scientist and ML Engineer at some companies to focus the latter on shipping production software using ML.
Time zones are an endless source of frustration, this one doesn’t sound too bad though:
https://github.com/LemmyNet/lemmy/pull/3496