This new data poisoning tool lets artists fight back against generative AI
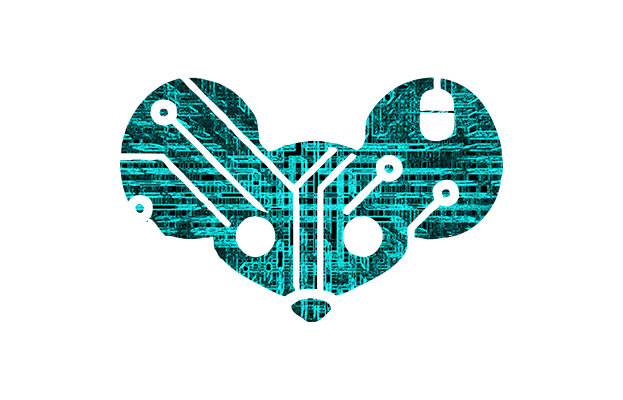
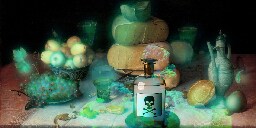
A new tool lets artists add invisible changes to the pixels in their art before they upload it online so that if it’s scraped into an AI training set, it can cause the resulting model to break in chaotic and unpredictable ways.
The tool, called Nightshade, is intended as a way to fight back against AI companies that use artists’ work to train their models without the creator’s permission.
[...]
Zhao’s team also developed Glaze, a tool that allows artists to “mask” their own personal style to prevent it from being scraped by AI companies. It works in a similar way to Nightshade: by changing the pixels of images in subtle ways that are invisible to the human eye but manipulate machine-learning models to interpret the image as something different from what it actually shows.
It's made by Ben Zhao? You mean the "anti AI plagerism" UChicago professor who illegally stole GPLv3 code from an open source program called DiffusionBee for his proprietary Glaze software (reddit link), and when pressed, only released the code for the "front end" while still being in violation of GPL?
The Glaze tool that promised to be invisible to the naked eyes, but contained obvious AI generated artifacts? The same Glaze that reddit defeated in like a day after release?
Don't take anything this grifter says seriously, I'm surprised he hasn't been suspended for academic integrity violation yet.
Thanks for added background! I haven't been monitoring this area very closely so wasn't aware, but I'd have thought a publication that has been would then be more skeptical and at least mention some of this, particularly highlighting disputes over the efficacy of the Glaze software. Not to mention the others they talked to for the article.
Figures that in a space rife with grifters you'd have ones for each side.
Don't worry, it is normal.
People don't understand AI. Probably all articles I have read on it by mainstream media were somehow wrong. It often feels like reading a political journalist discussing about quantum mechanics.
My rule of thumb is: always assume that the articles on AI are wrong. I know it isn't nice, but that's the sad reality. Society is not ready for AI because too few people understand AI. Even AI creators don't fully understand AI (this is why you often hear about "emergent abilities" of models, it means "we really didn't expect it and we don't understand how this happened")
Yeah, I view science/tech articles from sources without a tech background this way too. I expected more from this source given that it's literally MIT Tech Review, much as I'd expect more from other tech/science-focused sources, albeit I'm aware those require scrutiny just as well (e.g. Popular Science, Nature, etc. have spotty records from what I gather).
Also regarding your last point, I'm increasingly convinced AI creators' (or at least their business execs/spokespeople) are trying to have their cake and eat it too in terms of how much they claim to not know/understand how their creations work while also promoting how effective it is. On one hand, they genuinely don't understand some of the results, but on the other, they do know enough of how it works to have an idea of how/why those results came about, however it's to their advantage to pretend they don't insofar as it may mitigate their liability/responsibility should the results lead to collateral damage/legal issues.
By that logic humanity isnt ready for personal computers since few understand how they work.
Kind of true. Check the law proposals on encryption around the world...
Technology is difficult, most people don't understand it, result is awful laws. AI is even more difficult, because even creators don't fully understand it (see emergent behaviors, i.e. capabilities that no one expected).
Computers luckily are much easier. A random teenager knows how to build one, and what it can do. But you are right, many are not yet ready even for computers
I read an article the other day about managers complaining about zoomers not even knowing how type on a keyboard.
That was certainly true in the 90s. Mainstream journalism on computers back then was absolutely awful. I'd say that only changed in the mid-2000 or 2010s. Even today, tech literacy in journalism is pretty low outside of specialist outlets like, say, Ars.
Today I see the same thing with new tech like AI.
Oh, how I wish the FSF had more of their act together nowadays and were more like the EFF or ACLU.
You should check out the decompilation they did on Glaze too, apparently it's hard coded to throw out a fake error upon detecting being ran on an A100 as some sort of anti-adversarial training measure.
That's hilarious, given that if these tools become remotely popular the users of the tools will provide enough adversarial data for the training to overcome them all by itself, so there's little reason to anyone with access to A100's to bother trying - they'll either be a minor nuisance used a by a tiny number of people, or be self-defeating.
Thank you, Margot Robbie! I'm a big fan!
You're welcome. Bet you didn't know that I'm pretty good at tech too.
Also, that's Academy Award nominated character actress Margot Robbie to you!
Oh no, another complicated way to jpeg an image that an ai training program will be able to just detect and discard in a week's time.
They don't even need to detect them - once they are common enough in training datasets the training process will "just" learn that the noise they introduce are not features relevant to the desired output. If there are enough images like that it might eventually generate images with the same features.
Here's the paper: https://arxiv.org/pdf/2302.04222.pdf
I find it very interesting that someone went in this direction to try to find a way to mitigate plagiarism. This is very akin to adversarial attacks in neural networks (you can read more in this short review https://arxiv.org/pdf/2303.06032.pdf)
I saw some comments saying that you could just build an AI that detects poisoned images, but that wouldn't be feasible with a simple NN classifier or feature-based approaches. This technique changes the artist style itself to something the AI would see differently in the latent space, yet, visually perceived as the same image. So if you're changing to a different style the AI has learned, it's fair to assume it will be realistic and coherent. Although maaaaaaaybe you could detect poisoned images with some dark magic tho, get the targeted AI then analyze the latent space to see if the image has been tampered with
On the other hand, I think if you build more robust features and just scale the data this problems might go away with more regularization in the network. Plus, it assumes you have the target of one AI generation tool, there are a dozen of these, and if someone trains with a few more images in a cluster, that's it, you shifted the features and the poisoned images are invalid
Trying to detect poisoned images is the wrong approach. Include them in the training set and the training process itself will eventually correct for it.
Diffusion approaches etc. do not involve any conscious "building" of features in the first place. The features are trained by training the net to match images with text features correctly, and then "just" repeatedly predict how to denoise an image to get closer to a match with the text features. If the input includes poisoned images, so what? It's no different than e.g. compression artifacts, or noise.
These tools all try to counter models trained without images using them in the training set with at most fine-tuning, but all they show is that models trained without having seen many images using that particular tool will struggle.
But in reality, the massive problem with this is that we'd expect any such tool that becomes widespread to be self-defeating, in that they become a source for images that will work their way into the models at a sufficient volume that the model will learn them. In doing so they will make the models more robust against noise and artifacts, and so make the job harder for the next generation of these tools.
In other words, these tools basically act like a manual adversarial training source, and in the long run the main benefit coming out of them will be that they'll prod and probe at failure modes of the models and help remove them.
Just to start with, not very experienced with neural networks at all beyond messing with openCV for my graduation project.
Anyway, that these countermeasures expose "failure modes" in the training isn't a great reason to stop doing this, e.g. scammers come up with a new technique, we collectively respond with our own countermeasures.
If the network feedbacks itself, then cool! It has developed its own style, which is fine. The goal is to stop people from outright copying existing artists style.
It doesn't need to "develop its own style". That's the point. The more examples of these adversarial images are in the training set, the better it will learn to disregard the adversarial modifications, and still learn the same style. As much as you might want to stop it from learning a given style, as long as the style can be seen, it can be copied - both by humans and AI's.
There's a lot of interesting detail to your side of the discussion I may not yet have the knowledge of. How does the eye see? We find edges, gradients, repeating patterns which become textures, etc etc... But our systems can be misdirected, see the blue/yellow dress for example. NNsbhave the luxury of being rapidly iterated I guess, compared to our lifespans.
I'm asking questions I don't know answers to here: if the only source of input data for a network is subtly corrupted, won't that guarantee corrupted output as well? I don't see how one can "train out" the corruption which misdirects the network without access to some pristine data.
Don't get me wrong, I'm not naive enough to believe this is foolproof, but I do want to understand why this technique doesn't actually work, and by extension better understand how training a nn actually works.
We have to distinguish between different kinds of "corruption", here. What you seem to be describing is "if we only feed the model data from rule34, will it ever learn proper human anatomy" and the answer is no, it won't. You'll have to add data which narrows the range of body proportions from cartoonish to, well, real. That's an external source of corruption: Feeding it bad data (for your own definition of "bad"). Garbage in, garbage out.
The corruption that these adversarial models are exploiting though is inherent in the model they're attacking. Take... ropes and snakes and cats (or, generally, mammals). Good example: It is incredibly easy for a cat to mistake a rope for a snake -- it looks exactly the same to the first layers of the visual cortex and evolution would rather have the cat jump away as soon as possible than be bitten, and it doesn't hurt to jump away from a rope (even though the cat might end up being annoyed or ashamed (yes cats can 110% be self-conscious different story)), so when there's an unexpected wiggly shape the first layers directly tell the motor cortex to move, short-circuiting any higher processing.
That trait has been written into the network by evolution, very similar to how we train AI models -- conceptually, that is: In both cases the network gets trained for fitness for a purpose (the implementation details are indeed rather different but also irrelevant):
What those adversarial models do kinda looks like this: Take a picture of a rope. Now randomly shift pixels to make the rope subtly more snake-like until you get your cat to jump as reliably as possible, in as many different situations as possible, e.g. even if they're expecting it and staring straight at it. Sell the product for a lot of money. People start posting pictures of ropes, rope manufacturers adjust their weaving patterns. Other cats see those pictures and ropes, some jump, and others only feel a bit, or a lot, uneasy. The ones that jump will not be able to procreate, any more, being busy jumping, while the uneasy ones will continue to evolve. After a couple of generations no cat cares about those ropes with shifted pixels any more.
Whether that trains general immunity against adversarial attacks -- I wouldn't be so sure. It very likely will make the rope/snake distinction more accurate. But even if it doesn't build general immunity, it's an eternal cat and mouse game and no artist will be willing to continue paying for that kind of software when it's going to get defeated within days, anyway, because that's just how fast we can evolve models.
Oh. Back to the definition of corruption: If all the pictures of rope that our models ever see have shifted pixels then it's just going to assume that is the norm, and distinguish it from snakes because the tags say "rope" in one case, and "snake" in the other. The original un-shifted pictures probably won't be an adversarial attack because they're not a product of trying to get cats to jump.
Quick iteration is definitely the big thing. (The eye is fun because it's so "badly designed" - we're stuck in a local maxima that just happens to be "good enough" for us to not overcome the big glaring problems)
And yes, if all the inputs are corrupted, the output will likely be too. But 1) they won't all be, and as long as there's a good mix that will "teach" the network over time that the difference between a "corrupted cat" and an "uncorrupted cat" are irrelevant, because both will have most of the same labels associated with them. 2) these tools work by introducing corruption that humans aren't meant to notice, so if the output has the same kind of corruption it doesn't matter. It only matters to the extent the network "miscorrupts" the output in ways we do notice enough so that it becomes a cost drag on training to train it out.
But you can improve on that pretty much with feedback: Train a small network to recognize corruption, and then feed corrupted images back in as negative examples to teach it that those specific things are particularly bad.
Picking up and labelling small sample sets of types of corruption humans will notice is pretty much the worst case realistic effect these tools will end up having. But each such countermeasure will contribute to training sets that make further corruption progressively harder. Ultimately these tools are strictly limited because they can't introduce anything that makes the images uglier to humans, and so you "just" need to teach the models more about the limits of human vision, and in the long run that will benefit the models in any case.
Lol... I just read the paper, and Dr Zhao actually just wrote a research paper on why it's actually legally OK to use images to train AI. Hear me out...
He changes the 'style' of input images to corrupt the ability of image generators to mimic them, and even shows that the super majority of artists even can't tell when this happens with his program, Glaze... Style is explicitly not copywriteable in US case law, and so he just provided evidence that the data OpenAI and others use to generate images is transformative which would legally mean that it falls under fair use.
No idea if this would actually get argued in court, but it certainly doesn't support the idea that these image generators are stealing actual artwork.
So tl;dr he/his team did two things:
On the surface it sounds all good, but I can't help but notice a future conflict of interest for Zhao should Glaze ever become monetized. If it were to be ruled illegal to train AI on content without permission, tools like Glaze would be essentially anti-theft devices, but while it remains legal to train AI this way, tools like Glaze stand to perhaps become necessary for artists to maintain the pre-AI status quo w/r/t how their work can be used and monetized.
I am sure we already got a budget version of this called the jpeg.
Speaking of jpeg I miss the "needs more jpeg" bot that used to run on reddit, that shit was hilarious.
Reddit was Reddit for 18 fucking years. Just abandoning it leaves a massive hole. It’s gonna take a long time to fill it.
:(
It really will.
Saying that, fuck spez
So say we all:
Fuck /u/spez
New CAPCHA just dropped.
This is already a concept in the AI world and is often used while a model is being trained specifically to make it better. I believe it's called adversarial training or something like that.
No, that's something else entirely. Adversarial training is where you put an ai against a detector AI as a kind of competition for results.
Its called adversarial attack, this is an old video (5 years) explaining how it works and how you can potentially do it charging just one pixel on the image.
https://youtu.be/SA4YEAWVpbk?si=xObPveXTT2ip5ICG
Here is an alternative Piped link(s):
https://piped.video/SA4YEAWVpbk?si=xObPveXTT2ip5ICG
Piped is a privacy-respecting open-source alternative frontend to YouTube.
I'm open-source; check me out at GitHub.
"I can tell this is toxic by the pixels."
"We like to call them poison pixels."
I remember in the early 2010s reading an article like this one on openai.com talking about the dangers of using AI for image search engines to moderate against unwanted content. At the time the concern was CSAM salted to prevent its detection (along with other content salted with CSAM to generate false positives).
My guess is since we're still training AI with pools of data-entry people who tag pictures with what they appear to be, so that AI reads more into images than their human trainers (the proverbial man inside the Iron Turk).
This is going to be an interesting technology war.
Ooo, this is fascinating. It reminds me of that weird face paint that bugs out facial-recognition in CCTV cameras.
Or the patterned vinyl wraps they used on test cars that interferes with camera autofocus.
I am waiting for the day that some obsessed person starts finding ways to do like code injection in pictures.
Here ya go
Invisible changes to pixels sound like pure BS to me. I'm sure others know more about it than i do but I thought pixels were very simple things.
"Invisible changes to pixels" means "a human can't tell the difference with a casual glance" - you can still embed a shit-ton of data in an image that doesn't look visually like it's been changed without careful inspection of the original and the new image.
If this data is added in certain patterns it will cause ML models trained against the image to draw incorrect conclusions. It's a technical hurdle that will slow a casual adversary, someone will post a model trained to remove this sometime soon and then we'll have a good old software arms race and waste a shit ton of greenhouse emissions adding and removing noise and training ever more advanced models to add and remove it.
You can already intentionally poison images so that image recognition draws incorrect conclusions fairly easily, this is the same idea but designed to cripple ML model training.
You're right, in that pixels are very simple things. However, you and I can't tell one pixel from another in an image, and at the scale of modern digital art (my girlfriend does hers at 300dpi), shifting a handful of pixels isn't going to make much of a visible difference to a person, but a LLM will notice them.
An AI model will "notice them" but ignore them if trained on enough copies with them to learn that they're not significant.
LLM is the wrong term. That's Large Language Model. These are generative image models / text-to-image models.
Truthfully though, while it will be there when the image is trained, it won't 'notice' it unless you distort it significantly (enough for humans to notice as well). Otherwise it won't make much of a difference because these models are often trained on a compressed and downsized version of the image (in what's called latent space)
have you ever seen those composite images made by combining a huge number of other, radically different images in such a way that each whole image acts like one "pixel" of the overall image? i bet AI models 'see' those images very differently than we do.
Pixels are very simple things, literally 3-5 3 digit numbers.
But pixels mean little too a generative AI - it's all about relationship between pixels. All AI are high dimensional shapes right now... If you break up the shape strategically, it'll poison the image
Will this poison pill work? Probably, for at least a while...
A pixel has a binary representation. All of the significant bits for the pixel may not not be needed to display the color of that pixel so there is often excess that can be used or modified. A person wouldn’t see it but an AI reading just the binary would.
Obviously this is using some bug and/or weakness in the existing training process, so couldn't they just patch the mechanism being exploited?
Or at the very least you could take a bunch of images, purposely poison them, and now you have a set of poisoned images and their non-poisoned counterparts allowing you to train another model to undo it.
Sure you've set up a speedbump but this is hardly a solution.
No! It's not using an internal exploit, it's rather about finding a way to visually represent almost the same image, but instead using latent features with different artists (e.g, which would confuse a dreambooth+lora training), however, the method they proposed is flawed, I commented more on https://lemmy.world/comment/4770884
I'd assume the issue is that if someone tried to patch it out, it could legally be shown they were disregarding people's copyright.
It isn't against copyright to train models on published art.
The general argument legally is that the AI has no exact memory of the copyrighted material.
But if that's the case, then these pixels shouldn't need be patched. Because it wouldn't remember the material that spawned them.
Is just the argument I assume would be used.
It's like training an artist who's never seen a banana or a fire hydrant, by passing them pictures of fire hydrants labelled "this is a banana". When you ask for a banana, you'll get a fire hydrant. Correcting that mistake doesn't mean "undoing pixels", it means teaching the AI what bananas and fire hydrants are.
Well, I guess we'll see how that argument plays in court. I don't see how it follows, myself.
What is "patching pixels" and who would do it?
Is that not answered in the original article?
Explain
In order to violate copyright you need to copy the copyrighted material. Training an AI model doesn't do that.
Obviously, with so many different AIs, this can not be a factor (a bug).
If you have no problem looking at the image, then AI would not either. After all both you and AI are neural networks.
The neural network of a human and of an AI operate in fundamentally different ways. They also interact with an image in fundamentally different ways.
I would not call it “fundamentally” different at all. Compared to, say, regular computer running non-neural network based program, they are quite similar, and have similar properties. They can make a mistake, hallucinate, etc.
As a person who has done machine learning, and some ai training and who has a psychotic disorder I hate they call it hallucinations. It’s not hallucinations. Human hallucinations and ai hallucinations are different things. One is based of limited data , bias, or a bad data set with builds a fundamentally bad neural network connection which can be repaired. The other is something that can not be repaired, you are not working with bad data, your brain can’t filter out data correctly and you are building wrong connections. It’s like an overdrive of input and connections that are all wrong. So you’re seeing things, hearing things, or believing things that aren’t real. You make logical leaps that are irrational and not true and reality splits for you. While similarities exist, one is because people input data wrong, or because they cleaned it wrong, or didn’t have enough. And the other is because the human brain has wiring problem caused by a variety of factors. It’s insulting and it also humanizes computers to much and degrades people with this illness.
As I understand, healthy people hallucinate all the time, but in different sense, non-psychiatric sense. It is just healthy brain has this extra filter that rejects all hallucinations that do not correspond to the signal coming from reality, that is our brain performs extra checks constantly. But we often get fooled if we do not have checks done correctly. For example, you can think that you saw some animal, while it was just a shade. There is even statement that our perception of the world is “controlled hallucination” because we mostly imagine the world and then best fit it to minimize the error from external stimuli.
Of course, current ANNs do not have such extensive error checking, thus they are more prone to those “hallucinations”. But fundamentally those are very similar to what we have in those “generative suggestions” our brain generates.
Those aren’t quite the same as a hallucination. We don’t actually call them hallucinations. Hallucinations are a medical term. Those are visual disturbances not “controlled hallucinations”. Your brain filtering it out and the ability to ignore it makes it not a hallucination. It’s hallucinations in a colloquial sense not medical.
Fundamentally AI is not working the same, you are having a moment of where a process from when in the past every shadow was a potential danger so seeing a threat in the shadow first and triggering fight or flight is best for you as a species. AI has no fight or flight. AI has no motivation, AI just had limited, bad, or biased data that we put there and spits out garbage. It is a computer with no sentience. You are not really error checking, you are processing more information, or reassessing once the fight/flight goes down. AI doesn’t have more information to process.
Many don’t see people with psychotic disorders as equal people. They see them as dangerous, and and people to be locked away. They use their illnesses and problems as jokes and slurs. Using terms for their illness in things like this only adds to their stigma.
You are arguing about terminology use. Please google "controlled hallucinations" to see how people use the term in non-psychiatric way.
I know how it is used in a non psychiatric way, I brought that up it can be used in colloquially. That doesn’t diminish the way that it can be used to harm and stigmatize an already stigmatized group of people. There are other terms that can be used, but this is used because people want to humanize AI and do not care about dehumanizing people who have psychotic disorders.
The fact of the matter remains that AI creators are not people who specialize in human brains, but they act like computers and human brains are one and the same. Similarity doesn’t equal the same processes. They can choose different language but they do not. They could call it a processing error, a glitch, a distortion. All would be accurate, but no, they chose a term that is harmful to a minority group because no one cares about stigmatizing them.
Look at 2 and 3: https://www.merriam-webster.com/dictionary/hallucination
And I just do not see how that can stigmatize a group of people. It is like saying that the use of the word "headache" in non-medical contexts (e.g., "this homework is a headache") stigmatizes people with migraines. It just does not.
Listen, I live in a state where anyone who commits a violent crime, before they catch the person the police say, “he was hallucinating, they were hearing voices” aka mental illness is why they are doing this as a way to take away more rights. Also in this state if you are in a conservatorship for mental illness you legally are barred from voting. How can you say hallucination is not a loaded term? It is different from headache because people are not stigmatized for migraines. No one is taking away your voting rights for migraines. No one is saying you are a murderer for migraines.
You can use nearly any word in derogative sense so that it becomes offensive. "he had headache so strong, he went crazy". Context matters. And I personally do not even associate hallucination with mental illness. If anything, I associate it with psychedelics. Words are like tools - you can harm with them, but you can use them appropriately.
An AI don't see the images like we do, an AI see a matrix of RGB values and the relationship they have with each other and create an statistical model of the color value of each pixel for a determined prompt.
The AI can have some NaN, as a treat..
As a topping on some Pi
this is so dumb and clear it wont work at all. thats not the slightest how ai trains on images.
you would be able to get around this tool by just doing the nft thing and screenshot the image and boom code in the picture is erased.
What a dumb solution to a problem that doesn't need a solution. The problem isn't AI, it's the lack of understanding for the tech that has people thinking AI is theft.
Sorry "Artists" but I'm still working around your silly tools and generating beautiful AI Art 🥹
Makes porn